Text classification is the process of assigning a predefined category or label to sentences, paragraphs, textual reports, or other forms of unstructured text. The Text Analysis toolset in the GeoAI toolbox contains tools that use natural language processing (NLP) techniques for training text classification models as well as for using the models for classifying text.
The Train Text Classification Model tool trains NLP models for classifying text based on known classes or categories provided as part of a training dataset. Trained models can be used with the Classify Text Using Deep Learning tool to classify similar text into those categories.
Potential applications
The following are potential applications for this tool:
- Incomplete addresses can be classified by the country they belong to. This can help assign the appropriate locator to geocode those addresses more accurately.
- Geolocated tweets can be classified based on whether they indicate a sentiment for vaccine hesitancy. This can help identify areas where public education campaigns may increase public confidence and vaccine uptake.
- Categorizing the type of crime based on incident reports. This can help understand trends by aggregating crimes by their category and aid in planning remediation efforts.
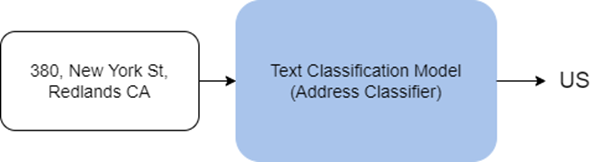
Text classification models in ArcGIS are based on the Transformer architecture proposed by Vaswani, et al. in the seminal “Attention is All you Need” paper. This allows the models to be more accurate and parallelizable, while requiring lesser labelled data for training.
Internally, text classification models in ArcGIS are deep neural networks that have two components:
- Encoder—The encoder serves as the model’s backbone and transforms the input text to a feature representation in the form of fixed-size vectors. The model uses well-known encoders such as BERT, ALBERT, and RoBERTa that are based on the transformer architecture and are pretrained on huge volumes of text.
- Classifier—A classifier serves as the model’s head and classifies the feature representations into multiple categories. A classifier is often a simple linear layer in the neural network.
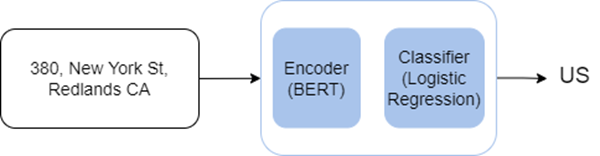
Text classification models in ArcGIS also support the Mistral model backbone. Mistral is a large language model based on a transformer architecture and operates as a decoder-only model. The key components of Mistral's architecture include the following:
- Sliding Window Attention—Efficiently handles long texts by processing them in smaller, overlapping segments, reducing computational cost and memory usage while preserving important context.
- Grouped Query Attention—Improves efficiency by clustering similar queries together, which minimizes the amount of attention computations and speeds up processing.
- Byte-Fallback BPE (Byte Pair Encoding) Tokenizer—Converts text into tokens for the model to process.
Note:
NLP models can be effective tools when automating the analysis of large volumes of unstructured text. As with other types of models, ensure that they are applied to relevant tasks with the appropriate level of human oversight and transparency about the type of model and training datasets used for training the model.
Use text classification models
The Classify Text Using Deep Learning tool can be used to apply a trained text classification model to unstructured text and categorize it into predetermined types. You can use pretrained text classification models from ArcGIS Living Atlas of the World or train custom models using the Train Text Classification Model tool.
The input to the Classify Text Using Deep Learning tool is a feature class or table containing the text to be classified. The input model can be an Esri model definition JSON file (.emd) or a deep learning model package (.dlpk). The model contains the path to the deep learning model file (containing model weights) and other model parameters. Certain models may have additional model arguments.
The tool creates a field in the input table containing the class or category label assigned to the input text by the model.
While the tool can run on CPUs, a GPU is recommended for processing as deep learning is computationally intensive. To run this tool using a GPU, set the Processor Type environment setting to GPU. If you have more than one GPU, specify the GPU ID environment setting instead.
Train text classification models
The Train Text Classification Model tool can be used to train NLP models for text classification. This tool uses a machine learning approach and trains the model by providing it with training samples consisting of pairs of input text and labelled classes. With the Mistral model backbone, it uses in-context learning, guiding the model's understanding and responses through input prompts and by providing the model with specific examples that help it infer the desired output. Training NLP models is a computationally intensive task, and a GPU is recommended for this.
The training data is provided in the form of an input table that contains a text field that acts as the predictor variable and a label field that contains the target class label for each input text in the table.
When training a text classification model, you can train the model from scratch, fine-tune a trained model, or use in-context learning. In general, language models using the transformer architecture are considered few-shot learners.
However, if you have access to a pretrained text classification model with the same set of target classes as there are in the training samples, you can fine-tune it on the new training data. Fine-tuning an existing model is often quicker than training a new model, and this process also requires fewer training samples. When fine-tuning a pretrained model, ensure that you keep the same backbone model that was used in the pretrained model.
The pretrained model can be an Esri Model Definition file or a deep learning package file. The output model is also saved in these formats in the specified Output Model folder.
Training deep learning models is an iterative process in which the input training data is passed through the neural network several times. Each training pass through the entire training data is known as an epoch. The Max Epochs parameter specifies the maximum number of times the training data is seen by the model while it is being trained. This is dependent on the model you are training, the complexity of the task, and the number of training samples. If you have a lot of training samples, you can use a small value. In general, it is a good idea to keep training for more epochs repeatedly, until the validation loss continues to go down.
The Model Backbone parameter specifies the preconfigured neural network that serves as the encoder for the model and extracts feature representations of the input text. This model supports BERT-, ALBERT-, and RoBERTa-based encoders that are based on the transformer architecture and are pretrained on large volumes of text in a semisupervised manner. These encoders have a good understanding of language and can represent the input text in the form of fixed length vectors that serve as inputs to the classification head of the model. The Model Backbone parameter also supports Mistral large language model (LLM). Mistral is a decoder-only transformer that uses Sliding Window Attention for efficient long-text processing, Grouped Query Attention to streamline computations, and Byte-Fallback BPE Tokenizer to handle diverse text inputs.
Model training occurs in batches, and the Batch Size parameter specifies the number of training samples that are processed for training at one time. Increasing the batch size can improve the performance of the tool. However, as the batch size increases, more memory is used. If an out of memory error occurs while training the model, use a smaller batch size.
The Learning Rate parameter is one of the most important hyperparameters. It is the rate at which the model weights are adjusted during training. If you specify a low learning rate, the model improves slowly and may take a long time to train, leading to wasted time and resources. A high learning rate may be counterproductive and may not learn well. With high learning rates, the model weights may be adjusted drastically, causing it to produce erroneous results. It is often best to not specify a value for the Learning Rate parameter, as the tool uses an automated learning rate finder based on the "Cyclical Learning Rates for Training Neural Networks" paper by Leslie N. Smith.
The tool uses part of the training data (10 percent by default) as a validation set. The Validation Percentage parameter allows you to adjust the amount of training data to be used for validation. For the Mistral model, at least 50 percent of the data must be reserved for validation, as Mistral requires a smaller training set and relies on a larger validation set to assess model performance.
By default, the tool uses an early stopping technique that causes model training to stop when the model is no longer improving over subsequent training epochs. You can turn this behavior off by unchecking the Stop when model stops improving parameter.
You can also specify whether the backbone layers in the pretrained model will be frozen, so that the weights and biases remain as originally designed. By default, the layers of the backbone model are not frozen, and the weights and biases of the Model Backbone value can be altered to fit the training samples. This takes more time to process but typically produces better results.
Text data often contains noise in the form of HTML tags and URLs. You can use the Remove HTML Tags and Remove URLs parameters to preprocess the text and remove the tags before processing.
References
Vaswani, Ashish, Noam Shazeer, Niki Parmar, Jakob Uszkoreit, Llion Jones, Aidan N. Gomez, Lukasz Kaiser, and Illia Polosukhin. "Attention Is All You Need." December 6, 2017. https://arxiv.org/abs/1706.03762.
Devlin, Jacob, Ming-Wei Chang, Kenton Lee, and Kristina Toutanova. "BERT: Pre-training of Deep Bidirectional Transformers for Language Understanding." May 24, 2019. https://doi.org/10.48550/arxiv.1810.04805.
"Encoder models." https://huggingface.co/course/chapter1/5?fw=pt.
Brown, Tom B. et al. "Language Models are Few-Shot Learners." July 22, 2020. https://doi.org/10.48550/arxiv.2005.14165.
Smith, Leslie N. "Cyclical Learning Rates for Training Neural Networks." April 4, 2017. https://doi.org/10.48550/arxiv.1506.01186.
"Mistral." https://docs.mistral.ai/getting-started/open_weight_models/.